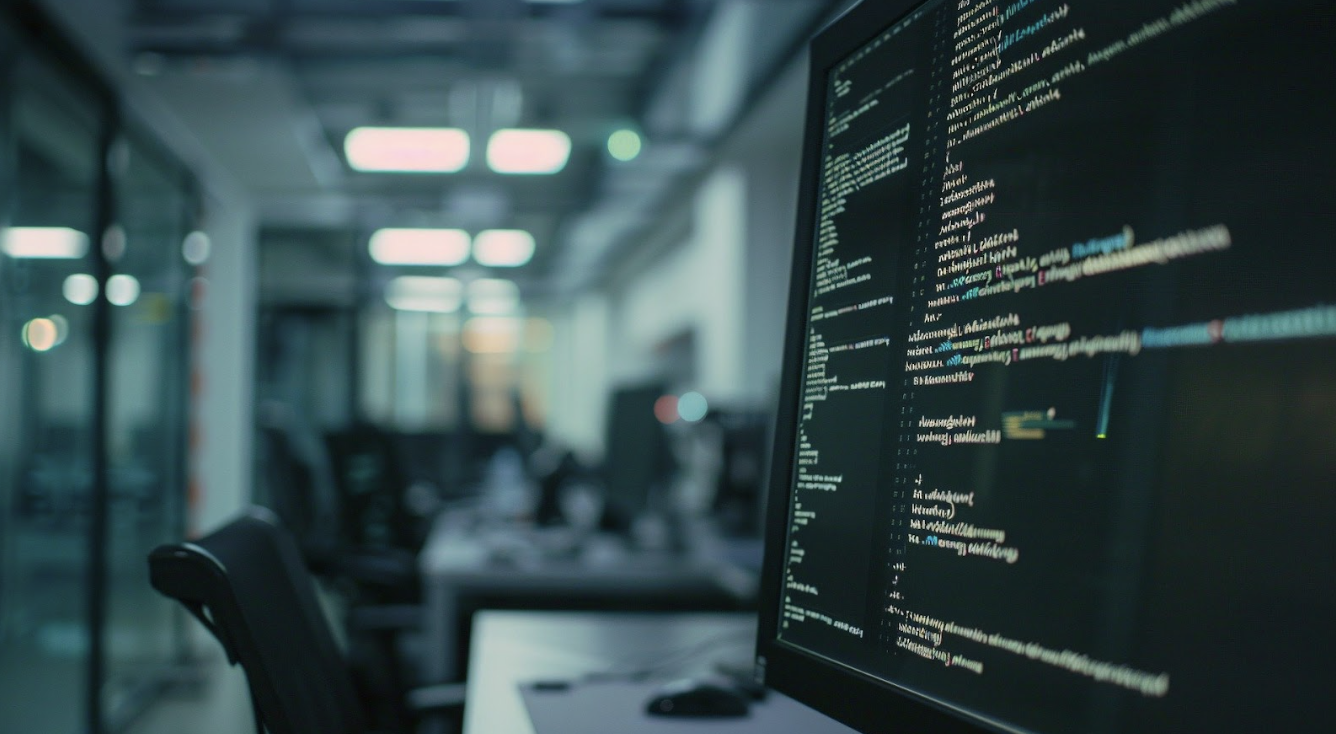
AI and Machine Learning are transforming the way businesses operate, especially in IT projects. In fact, the global annual revenue of the AI software market is approximately $100 billion. These technologies are no longer just buzzwords, they’re powerful tools that, when used effectively, can lead to breakthroughs in efficiency, decision-making, and innovation. But the key to unlocking their potential lies in understanding how to use them wisely. Whether you’re working on a small project or managing large-scale operations, knowing the best ways to leverage AI and ML can make a world of difference. Through this blog post, we aim to enlighten you with the top machine learning and AI integration tips.
How to Best Leverage AI and Machine Learning in Your IT Projects
Finding the Right Fit for AI and ML ⇢
Not every challenge in your IT project needs AI or ML. Sometimes, simpler solutions work just fine. But when you do decide to use these technologies, it’s important to be strategic and find the right ways to leverage AI and ML. Think about where AI and ML can truly make an impact.
For example:
Predicting Future Trends: Machine Learning is great at analyzing past data to forecast what might happen next. This can be invaluable in areas like sales, customer behavior, or even system maintenance.
Automating Repetitive Tasks: AI can handle routine tasks, allowing your team to focus on more creative and complex problems. Imagine freeing up hours of manual work just by automating processes.
Boosting Security: AI can spot unusual patterns that could indicate security threats. It’s like having an extra pair of eyes that never blink.
Building a Strong Data Foundation ⇢
Data is the fuel that powers AI and ML. But not just any data; good data. If you want these technologies to work for you, you need to start with a solid foundation.
Collecting the Right Data: Gather data that’s relevant to the problems you’re trying to solve. The more accurate and comprehensive your data, the better your AI and ML models will be.
Keeping Data Safe: With great data comes great responsibility. Ensure your data is protected and that you’re complying with privacy regulations. This isn’t just about avoiding fines—it’s about building trust with your customers and stakeholders.
Cleaning and Preparing Data: Raw data often contains errors, duplicates, or irrelevant information. Cleaning it up is crucial because even the most sophisticated AI models can’t perform well if the data they’re trained on is flawed.
Choosing the Right Tools and Platforms ⇢
When it comes to AI and ML, the tools you choose can make or break your project. There are countless options out there, from open-source libraries to fully managed platforms.
Open-Source Tools: Platforms like TensorFlow and PyTorch are popular because they’re flexible and powerful. TensorFlow is great for large-scale projects, while PyTorch is user-friendly and excellent for research and development.
Commercial Platforms: If you’re looking for something more out-of-the-box, platforms like IBM Watson or Google AI Platform offer pre-built models and services that can be integrated into your existing systems with relative ease.
Encouraging Collaboration ⇢
AI and ML projects often require people from different disciplines to work together. Data scientists, IT professionals, and business experts all bring something valuable to the table. For your project to succeed, fostering a culture of collaboration is key.
Bringing Teams Together: Cross-functional teams ensure that everyone’s expertise is leveraged. IT teams understand the technical requirements, while business experts provide insights into the practical applications.
Ongoing Learning: AI and ML are fast-evolving fields. Encourage continuous learning within your team so they can stay up-to-date with the latest developments.
Working in Iterations: Using an agile approach can be beneficial in AI projects. It allows your team to develop models in small, manageable steps, getting feedback and making improvements along the way.
Being Mindful of Ethics ⇢
AI is powerful, but it’s also a tool that can have significant consequences. Using AI ethically is not just about avoiding negative outcomes—it’s about actively working to make sure your technology benefits everyone.
Avoiding Bias: AI models can inherit biases from the data they’re trained on. It’s important to check for and mitigate these biases to ensure your AI applications are fair.
Transparency: Make your AI models understandable, especially to those who aren’t tech-savvy. People need to trust that AI isn’t a “black box” making decisions without human oversight.
Taking Responsibility: Clearly define who is accountable for AI-driven decisions. This can prevent problems down the line and ensure that your AI implementations are responsible and well-managed.
Integrating AI and ML Seamlessly ⇢
Introducing AI and ML into your existing systems can be challenging, but it’s crucial to maximize their potential and find the right ways to leverage machine learning and AI. The key is to integrate them in a way that enhances, rather than disrupts, your current processes.
Using APIs: Many AI platforms offer APIs that allow you to integrate AI capabilities into your existing systems without a complete overhaul. This makes the transition smoother and more manageable.
Adopting Microservices: If your IT environment is flexible, consider using a microservices architecture. This allows different parts of your system to evolve independently, making it easier to incorporate AI and ML where needed.
Leveraging Data Lakes: Storing large volumes of raw data in a data lake can make it easier to feed your AI and ML models with the information they need, without bogging down your system.
Measuring Success ⇢
Once your AI and ML systems are up and running, how do you know they’re working? Measuring success is about more than just technical performance; it’s about seeing real-world impact.
Model Performance: Track how well your models are performing using metrics like accuracy, precision, and recall. These numbers can tell you whether your AI is hitting the mark.
Business Outcomes: Beyond the tech metrics, look at how AI is affecting your business. Are you seeing improvements in efficiency, customer satisfaction, or revenue? These are the signs that AI is truly delivering value.
Ongoing Monitoring: AI models can degrade over time as data changes. Continuous monitoring allows you to spot issues early and update your models as needed.
Scaling AI Across Your Organization ⇢
Once you’ve successfully implemented AI in one area, the next step is scaling it across your organization. This is where the real transformation happens, but it requires careful planning.
Centralize Your Strategy: Develop a clear AI strategy that outlines how you plan to expand AI across the organization. This prevents silos and ensures everyone is working towards the same goals.
Create Centers of Excellence: Establish AI Centers of Excellence to provide support, best practices, and expertise across the organization. These hubs can drive consistency and innovation.
Automate and Standardize: As you scale, look for opportunities to automate repetitive tasks and standardize processes. This makes it easier to roll out AI solutions quickly and efficiently.
Preparing for the Future ⇢
AI and ML are dynamic fields, constantly evolving with new techniques and regulations. To keep up, you need to future-proof your AI investments.
Embrace Innovation: Encourage your team to explore new AI techniques and be open to experimentation. The next big breakthrough might be just around the corner.
Stay Compliant: Regulations around AI and data privacy are always changing. Make sure your AI initiatives are flexible enough to adapt to new rules and standards.
Invest in Scalable Infrastructure: As your AI needs to grow, so should your infrastructure. Consider cloud-based solutions that can scale with you, ensuring you’re always ready for what’s next.
Why Choose Blue Coding To Outsource For Manage AI and Machine Learning Projects
Blue Coding offers more than just outsourcing; we provide a partnership grounded in expertise and reliability for managing AI and Machine Learning projects. Our team of skilled professionals brings a ton load of experience to every project, ensuring that each solution is precisely aligned with your business goals. We focus on delivering high-quality results by using the latest technologies and best practices. With Blue Coding, you’ll have a dedicated partner committed to helping you use the power of AI and Machine Learning, driving your business forward in today’s competitive landscape. To learn more about how we can help with your specific goals, contact us now and schedule a strategy call!