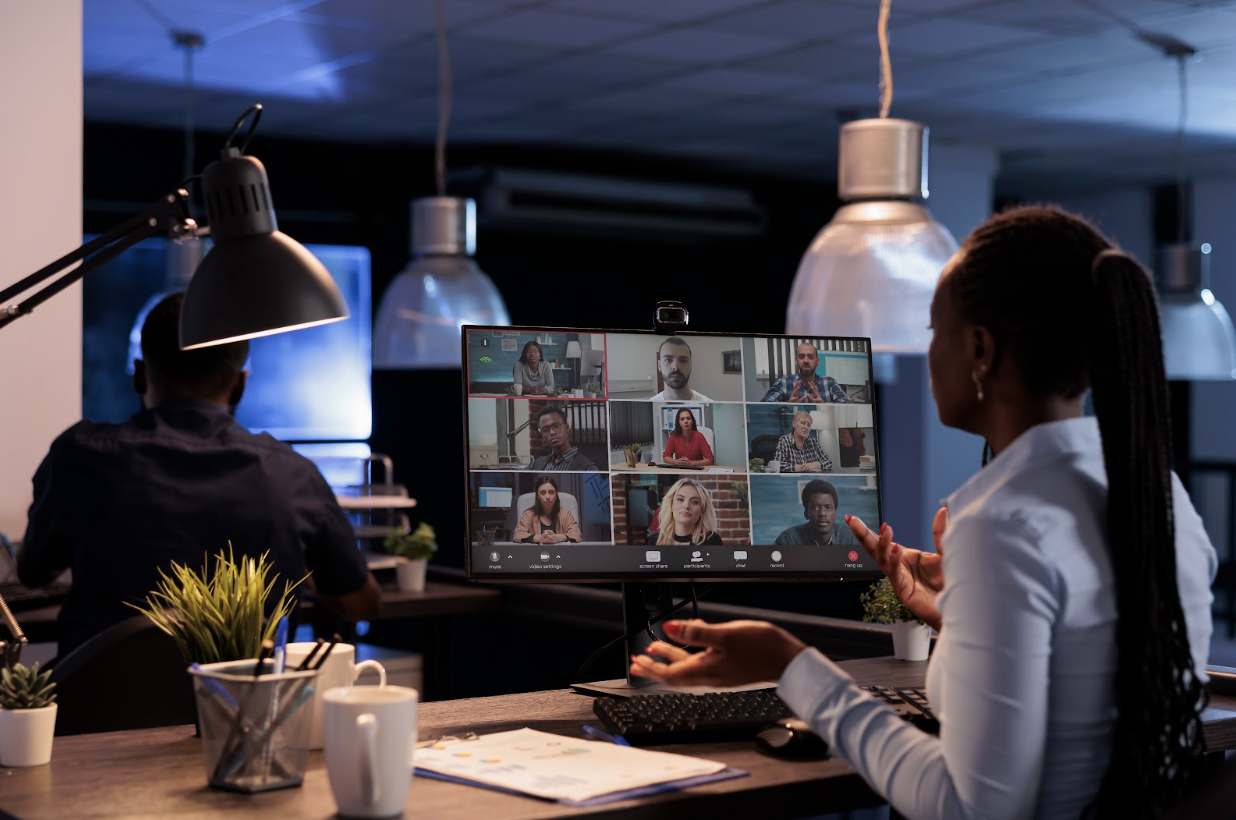
Machine learning outsourcing involves hiring external experts or firms to develop and implement machine learning solutions for a company. This approach is typically chosen by businesses that lack the in-house expertise or resources needed to handle complex machine learning projects. By outsourcing, companies can access specialized skills and advanced technologies, often leading to more efficient and cost-effective outcomes. The outsourcing process includes identifying suitable vendors, defining project requirements, and collaborating closely with the external team to ensure the solutions align with the business goals. In this blog post, we explore how, as a business, you can start outsourcing your machine learning requirements.
Things To Keep In Mind When Outsourcing Machine Learning Development Needs
Assess Your Needs and Objectives
Before embarking on machine learning (ML) outsourcing, clearly define your goals and the specific outcomes you want to achieve. Determine the type of ML project you need, such as predictive analytics, natural language processing, or computer vision. Evaluate the scope and complexity of the project to ensure you understand the level of expertise required. Identifying your needs helps in selecting the right outsourcing partner and sets a clear direction for the project.
Research Potential Partners
Conduct thorough research to find potential outsourcing machine learning developers. Look for firms or freelancers with a proven track record in machine learning. Evaluate their previous projects, client testimonials, and case studies to gauge their expertise. Consider their technical skills, including familiarity with relevant ML algorithms, programming languages like Python and R, and tools such as TensorFlow or PyTorch. Additionally, assess their domain expertise to ensure they understand the specific industry challenges and requirements.
Evaluate Technical and Cultural Fit
Ensure that the outsourcing partner aligns with your technical and cultural expectations. Conduct interviews and technical assessments to evaluate their skills and problem-solving capabilities. Assess their communication style, project management approach, and willingness to collaborate. A good cultural fit ensures smooth interactions and a more productive partnership. You should also verify their ability to understand and adapt to your company’s processes and workflows.
Define Clear Project Specifications
Create a detailed project specification document outlining the objectives, requirements, deliverables, and timelines. Clearly define the expected outcomes, data requirements, and performance metrics. Establish milestones and review points to monitor progress. This document serves as a reference point throughout the project, ensuring both parties have a mutual understanding of the project's scope and expectations.
Establish Communication Channels
Set up effective communication channels to facilitate regular updates and feedback. Decide on the frequency and mode of communication, such as weekly meetings, email updates, or project management tools like Jira or Trello. Clear communication helps in promptly addressing any issues and ensuring that the project stays on track. Establishing a single point of contact on both sides can streamline communication and reduce misunderstandings.
Set Up Data Security and Privacy Measures
Data security and privacy are critical when outsourcing ML projects. Ensure that the outsourcing partner complies with relevant data protection regulations, such as GDPR or CCPA. Establish clear protocols for data handling, storage, and access. Use secure methods for data transfer and anonymize sensitive information where possible. Draft a comprehensive non-disclosure agreement (NDA) to protect intellectual property and confidential data.
Implement Quality Assurance Processes
Define quality assurance (QA) processes to ensure the project meets the desired standards. Set up a robust testing framework to evaluate the ML models' performance and accuracy. Conduct regular code reviews, performance testing, and validation against the defined metrics. Continuous monitoring and iteration help in identifying and resolving issues early, ensuring the final deliverable is reliable and effective.
Plan for Integration and Deployment
Prepare for the integration and deployment of the ML solution within your existing systems. Work with the outsourcing partner to ensure the solution is compatible with your infrastructure. Plan for a phased deployment to minimize disruptions. Establish procedures for monitoring and maintaining the ML models post-deployment, including updating the models as new data becomes available and retraining them to improve performance over time.
Review and Reflect
After project completion, conduct a comprehensive review to evaluate the outcomes and the outsourcing process. Assess whether the project met its objectives and delivered the expected value. Gather feedback from all stakeholders to identify areas of improvement for future projects. Reflecting on the experience helps in refining your approach to machine learning outsourcing and building stronger partnerships in the future.
Methods for Starting Machine Learning Outsourcing in 2024
Hiring Freelancers
Collaborating with Consulting Firms
Utilizing Managed Services Providers (MSPs)
Academic and Research Collaborations
Involving Cloud Service Providers
Crowdsourcing Platforms
Building Remote Teams
Engaging Independent Consultants
Blue Coding - A Nearshore Outsourcing Agency
Blue Coding is a specialized nearshore software development agency that offers clients the help they need to hire remote developers, along with additional IT solutions like technical staff augmentation, custom app and web development, and IT consultations. Our experts work closely with clients, maintaining constant communication to ensure the best results, as customer satisfaction is our highest priority. To learn more about our services and features, contact us today and book your free discovery call now!